Optical Character Recognition: The Most Useful Innovation You Don't Think About
- Me
- Apr 20, 2023
- 5 min read
Updated: Jun 3, 2023
Editor's Note: This article was created as part of my fractional/interim role leading Growth and Demand Gen at Quandri. You can read more about my time there here.
Intro
Optical Character Recognition (OCR) is a software technology capable of capturing text elements from images or documents and converting them into machine-readable text format. OCR has been used in various industries since the 1950s, such as banking and healthcare, to help automate mundane data entry tasks. OCR has changed your life and you probably don’t even notice it. OCR is the central actor in software text recognition. Google Drive, depositing a check via your phone, Adobe Acrobat, Google Translate, barcode scanners, etc.
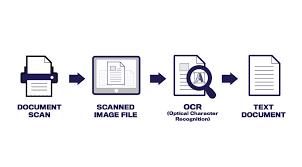
History
If you’re thinking this seems like a modern day invention, I did too. Algorithms, computer vision, digitizing physical assets, software - how old can it be? As it turns out, pretty old. OCR software and picture-to-text technology have been around since at least the late 1920s. An Austrian engineer named Gustav Tauschek patented an optical character recognition device in Germany in 1929 and again in the United States in 1935.
A brief tangent for history lovers – In addition to the image to text invention, Gustav developed 169 patents and sold them all to IBM. Given a five-year contract by the software giant, Tauschek used OCR technology to develop a punchcard-based accounting system.
In 1931, OCR technology was used in the creation of a text-to-telegraph device. 20 years later, a text-to-Morse Code device. In 1966, OCR became capable of reading handwriting and transforming it into text. Researchers began experimenting with ways to enable computers to scan printed text and read and interpret the data. One of the first attempts was made by the Royal Canadian Navy, when they used OCR to automatically read and sort incoming mail. In 1960s, the US Air Force developed a system called “OCR-A”, which enabled computers to automatically read and interpret text on documents. This system was adopted by the US Department of Defense and represents the first days of Spring for OCR technology.
Then in the 1970s, the OCR wagon found it’s wheels. Ray Kurzweil founded Kurzweil Computer Products, Inc., which developed the first commercial omni-font optical character recognition (OCR) product. Kurzweil’s product was capable of recognizing text printed in virtually any font. He then created a reading machine that could read text aloud in a text-to-speech format, which was ideal for those with vision impairments.
By the 1980s, OCR technology was an integral part of the economy - barcode scanners in retail stores, Xerox machines in offices and schools, scanning business documents into databases, etc. The technology and use cases have advanced since then, primarily in accuracy, processing power, and recognition formats. Today, OCR technology is used to transform text into digital data that can be manipulated and analyzed. It is used in a wide range of applications such as document scanning, document processing, archiving and retrieval, business process automation, data entry automation, and automation of back-office operations.
How the technology works
Computer vision is a broad field of study that uses algorithms and techniques to gain understanding from digital images or videos. OCR is a specific subset of computer vision designed to process text from images and videos. Put simply, OCR is a way to “read” characters from paper or other physical documents, such as photos or scans, and transform them into machine-readable text that can be edited, stored, and searched.
OCR works by breaking down an image into individual characters and mapping them to the corresponding digital character. The digital characters are converted into editable text that can be stored, manipulated and analyzed.
This process is similar to how a human interprets a document. First we localize the information, detect words and sequences, then use semantic knowledge to make sense of the information. We rely on the semantic knowledge to guide the text recognition - if a word is missing, we’re able to fill in the gap by predicting the most likely word based on our past experience. OCR works pretty much the same way.
First, it detects objects in the image and places boxes around the object’s position.
Then it detects text objects, looking for chains of characters in the image.
Each object is represented by a set of coordinates which is the bounding box that includes the object we are looking for (words in the above case).
So the features and approximate location of those features are extracted. An operation called region pooling resizes the objects to fit into the matrix. Now, we can get to work processing and understanding the text. The software runs through a set of tasks such as;
Word or image?
Object classification - think captcha test, is it an animal or plant?
Text recognition (character sequence to output text)
There’s more steps and nuance, but that’s the gist of how OCR works in practice. Two recent advancements are particularly promising and open up a new chapter in the story:
Natural language processing (NLP)
NLP enables the recognition and ability to extract meaning from text.
This makes it possible to analyze the content of documents and assign them structured tags.
Which provides valuable information for business processes such as document scanning, document indexing and document retrieval.
Artificial intelligence (AI) and machine learning (ML)
These enable the ability to identify text from images that are blurred, skewed or have low-quality resolution.
Also, AI and ML are used automatically detect and correct errors in text recognition - increasing both accuracy and speed.
Applications In Insurance
The insurance industry is undergoing rapid digital transformation, accelerated during COVID. The increase in AI and automation technology continue to spur this trend forward. OCR is one of the protagonists in this story. For many insurers, OCR is an invaluable part of a modern toolbox.
The biggest area OCR has changed the game is the claims process. Brokerages save time and money using OCR to scan and process documents. Additionally, OCR is used to customer verification, quote generation, and fraud detection.
The second area is improving customer service. Brokerages use OCR to automatically populate information into forms, saving time for customers and those servicing them. Data capture and matching via OCR quickly locates customer records in databases. And nothing’s worse for customers than providing the same information over and over again. Customers expect you to remember what they’ve told you.
The third area we’ll mention here is managing risk. Insurers use OCR to analyze large amounts of data, insurers can identify trends and patterns that may indicate potential risks. OCR helps monitor claims activity and flag suspicious claims.
Additionally, OCR reduces manual errors, which may not fall under the Risk Management department, but do represent business risk. Human error is inevitable. Manual, repetitive data entry and transfer always increase the error rate. This article in the Journal of Applied Sciences showed repetition leads to fatigue and fatigue leads to increased error rates. While not all OCR variations are more accurate than humans, some are much more accurate - and all variations are more predictable and repeatable.
Last, but not least (for your staff) is administrative automation. Brokerages have a lot of admin processes. Onboarding customer accounts, fighting a battle with your BMS, entering information into forms, etc. OCR, within an intelligent automation tool, can take on much of this work. Repetitive tasks are highly correlated with lower employee satisfaction, engagement, and retention. Hiring enough great talent is hard enough. Hiring enough great talent while your losing great talent is almost impossible.
Comments